- Home
- Deputy governors' speeches
- Artificial intelligence: A blessing or a...
Artificial intelligence: A blessing or a curse in the financial sector transformation?
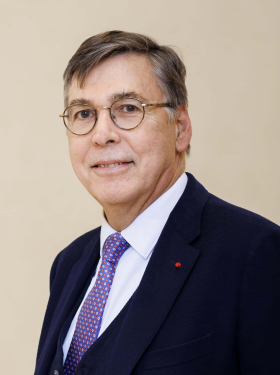
Denis Beau, First Deputy Governor of the Banque de France
Published on the 8th of November 2024
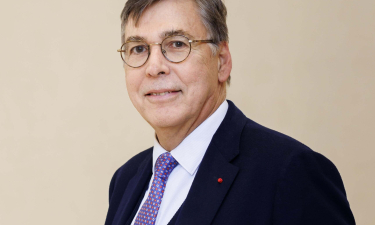
Denis Beau's speech
First Deputy Governor of the Banque de France
Singapore, 8 November 2024
Ladies and gentlemen,
Let me start with stating the obvious: the use of Artificial intelligence (AI) is on the rise. Indeed, the possibilities offered by this set of technologies seem immense. AI has underpinned such progress that this year the Nobel Prize in Physics was awarded to two pioneers of artificial neural networks, while the Nobel Prize in Chemistry recognized an application of AI to understand the structure of proteins.
In the financial sector, too, AI is increasingly used, for example to assess credit risk, set insurance rates, or estimate asset volatility. This technology is now the main driver of the digital transformation of the financial sector. And this transformation obviously cannot leave the central banker and financial supervisor that I am unmoved. It raises the question of the opportunities and risks of these changes for the financial sector (I). And it also raises questions for central banks and financial supervisors about their own operations and their mastery of these new technologies: how will we monitor the use of AI by financial players in the future, and what are the possibilities offered by these technologies for our own activities? (II) Let me address those issues briefly and in turn.
I/ On the first issue of opportunities and risks, let me start with this initial observation to put it in the right perspective: AI, coupled with data science, is a powerful force driving the transformation of the financial sector.
1/ Our observations show that AI is increasingly used by financial institutions, in all segments of the value chain. Banks use these tools to improve the “user experience”, but also to automate and optimize a number of internal processes. AI is also used to monitor and control risks, as demonstrated by its success in use cases relating to the fight against fraud or money laundering and the financing of terrorism (AML/CFT).
The advent of generative AI over the past two years has been a powerful force accelerating already underway trends. Indeed, it has led to a revolution in the accessibility of AI technologies: the ability to interact in natural language with algorithms - via large language models or LLMs - has made the adoption of new technologies much easier. It is also accelerating innovation momentum within companies, as code writing is no longer confined to IT staff. AI could thus have a powerful impact on productivity: in France, the AI Commission, chaired by Anne Bouverot and Philippe Aghion, estimated at around 1% per year the additional growth that AI deployment could generate in our country over the next ten years.
This technological revolution allows many use cases to develop. For example, generative AI-powered chatbots have the ability to provide a personalized response that is more tailored to the user’s situation. In insurance, satellite image processing can in some cases be used to directly assess the damage caused to a building, and then automatically manage customer compensation. And this is just the beginning: we are probably far from having explored all the possibilities these technologies can provide.
Well-controlled AI can therefore increase the efficiency of financial institutions and contribute to enhance their revenues, thereby weighting positively on their profitability - which is a central element of their soundness - including by providing them with risk control solutions.
2/ However, the medal has a flipside, and the power of the solutions developed comes with significant risks. I would like to mention two of them here.
First, there is the risk that these technologies may be misused. The complexity and novelty of some modeling approaches can indeed lead to more errors in either design or use of the systems. This poses a risk not only to customers but also to the financial health of institutions, since the wrong calibration of a model could lead to systematic losses. Two elements reinforce these risks. First, the real-time adjustment of the parameters of certain models, which is their strength, can also result in rapid drift. Second, some AI systems are particularly opaque, giving rise to a “black box” phenomenon. This is of course a customer protection issue, because customers need to be able to understand automated decisions made about them. But it is also a governance issue: an institution that does not have a good understanding of the decisions made by its AI systems cannot claim to control the risks associated with them.
I would like to mention a second risk, and not the least important: the cyber risk. In recent years, it has become the number one operational risk in the financial sector. AI can amplify this risk, particularly because technology greatly increases the dangerousness of attackers, such as sneaky code-writing assistants to design malware and synthetic voices to facilitate impersonation. The list of threats is long. This is one of the reasons behind the European DORA Regulation, which will come into force in January 2025.
However, technology can also be mobilized to counter these attacks. AI is thus a double-edged tool, as the field of payments illustrates perfectly. In payments, AI can significantly facilitate scams, for instance through deepfakes. But it is also likely to become a key ally in the fight against fraud, helping to identify fraud patterns more efficiently and quickly. In a way, this is a tale of “Catch me if you can” - to refer to a movie well known to payment experts - where fraudsters and authorities resort to increasingly sophisticated tactics to outwit each other.
As part of its legal mandate to ensure the security of cashless payment instruments, the Banque de France has been spearheading efforts to assess the implications of AI for payment security, through the Observatory for the Security of Means of Payment (OSMP), which I have the privilege to chair. Advanced scoring tools have been used for years to mitigate fraud on card-based payments. These tools continue to be improved, leveraging specifically on strong authentication protocols such as 3-D Secure. While instant payments are expanding rapidly, I believe the time has come to enrich those tools with the use of AI to secure other payment channels such as credit transfers, direct debits and money remittances.
II/ But the technological revolution generated by AI is also a driving force for the transformation of financial authorities, with a dual movement: they must both prepare to supervise the use of AI by the financial sector, and use the new technologies to improve their efficiency and develop new capabilities.
1/ In order to address AI risks and enable the financial sector to take full advantage of these opportunities, we need to build effective regulation. The move toward a regulatory framework has already begun: with its AI Act, which came into force this summer, the European Union has adopted the world’s first legal framework and has laid the foundations for “trustworthy AI”. To achieve this, the regulation distinguishes between several risk levels, within which “high risks” - which form the core of the text - will apply to the financial sector in at least two respects: creditworthiness assessment when granting credit to natural persons; risk assessment and pricing in health and life insurance.
For financial sector use cases, the AI Act entrusts the role of “market surveillance authority” to the national financial supervisors - and therefore in France to the ACPR, the prudential supervisory and resolution authority. This is a wise choice! It will enable the implementation of this text to be coordinated as closely as possible with existing sectoral rules, with the help - when the time comes - of guidelines from the European supervisory authorities.
The ACPR stands ready to play the new role that the AI Act will entrust to it. It will do so by using a “risk-based” approach, and by making maximum use of existing synergies with prudential supervision, in order to limit the additional burden for financial institutions and for itself.
However, I am not minimising the challenge that the implementation of the AI Act represents: beyond organisational aspects, we will need to develop an AI audit methodology, which undoubtedly represents a quantum leap in our working methods. We welcome this challenge not as a constraint, but as an opportunity: everywhere in the world, indeed, regardless of whether a specific regulation has been adopted, financial supervisors need to develop new capabilities in order to respond to the growing use of AI by financial actors. And, in fact, we intend to build effective cooperation with other supervisors, particularly at European and international level.
2/ We also want to use AI technologies for our own purposes. We intend to improve our operational efficiency, while giving the Banque de France and ACPR staff new capabilities. Indeed, we want to build a trustworthy AI framework, in which technology complements rather than replaces humans.
Investment in AI is therefore addressed in a specific action in our strategic plan, monitored at the highest level. This action has two main ambitions: generate a range of generic online services for all our agents - for example with enhanced search capabilities, or document analysis and synthesis functions - and provide support AI-agents to address more specific business needs.
Because learning by doing is the best way to progress, it will start with 5 priority use cases in the short run. To illustrate, let me mention three of them: an internal chatbot assistant to answer employees’ questions on HR issues, a tool to detect atypical transactions for AML/CTF purposes - particularly in the French Treasury transactions managed by the Banque de France - and a structured financial product mapping tool for the ACPR.
One last point to conclude: the deployment of AI at the Banque de France and the ACPR is being done in a reasoned and controlled manner. For example, we only use public cloud infrastructure when processing non-sensitive data. More generally, we ensure that we have a thorough understanding of the models and their results, in order to maintain full control over our activities. And we are gradually deploying and integrating AI, making room for training, feedback and continuous improvement.
Thank you for your attention.
Download the full publication
Updated on the 8th of November 2024